Pricing Insurance Risk
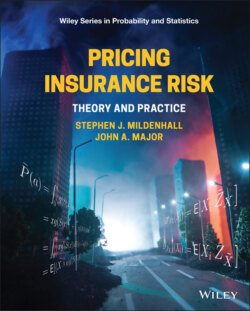
Реклама. ООО «ЛитРес», ИНН: 7719571260.
Оглавление
Stephen J. Mildenhall. Pricing Insurance Risk
Pricing Insurance Risk. Theory and Practice
Contents
List of Figures
List of Tables
Guide
Pages
Preface
1 Introduction
1.1 Our Subject and Why It Matters
1.2 Players, Roles, and Risk Measures
1.3 Book Contents and Structure
1.3.1 Part I: Measuring Risk
1.3.2 Part II: Portfolio Pricing
1.3.3 Part III: Price Allocation
1.3.4 Part IV: Advanced Topics
1.3.5 Further Structure
1.4 What’s in It for the Practitioner?
1.5 Where to Start
2 The Insurance Market and Our Case Studies
2.1 The Insurance Market
2.2 Ins Co.: A One-Period Insurer
2.3 Model vs. Reality
2.4 Examples and Case Studies
2.4.1 The Simple Discrete Example
Exercise 1
2.4.2 Tame Case Study
2.4.3 Catastrophe and Non-Catastrophe Case Study
2.4.4 Hurricane and Severe Storm Case Study
2.4.5 Computational Methods
2.5 Learning Objectives
3 Risk and Risk Measures
3.1 Risk in Everyday Life
3.2 Defining Risk
3.3 Taxonomies of Risk
3.3.1 Diversifiable Risk
3.3.2 Systemic Risk
3.3.3 Types of Uncertainty
3.4 Representing Risk Outcomes
3.4.1 Explicit Representation
3.4.2 Implicit Representation
3.4.3 Dual Implicit Representation
3.4.4 Dictionary between the Three Representations
3.5 The Lee Diagram and Expected Losses
3.5.1 The Lee Diagram
3.5.2 Expected Losses and the Lee Diagram
3.5.3 Layer Notation
3.5.4 Computing Layer Losses with the Lee Diagram
3.5.5 Algorithm to Evaluate Expected Loss for Discrete Random Variables
3.6 Risk Measures
3.6.1 Risk Preferences and Risk Measures
3.6.2 Volume, Volatility, and Tail Risk
3.6.3 Applications of Risk Measures
3.6.4 Risk Measure Functional Forms
3.7 Learning Objectives
4 Measuring Risk with Quantiles, VaR, and TVaR
4.1 Quantiles
4.1.1 Definition and Examples
4.1.2 Algorithm to Compute Quantiles
4.1.3 Simulation Using Quantile Functions
4.2 Value at Risk. 4.2.1 Motivation
4.2.2 Definition and Examples
4.2.3 Return Periods
4.2.4 Aggregate and Occurrence Probable Maximal Loss and Catastrophe Model Output
4.2.5 The Failure of VaR to be Subadditive
4.2.6 Worst-Case VaR and the Rearrangement Algorithm
The Rearrangement Algorithm
4.2.7 When Is VaR Subadditive?
4.3 Tail VaR and Related Risk Measures. 4.3.1 Motivation
4.3.2 Definition of TVaR
4.3.3 TVaR of a Normal Random Variable
4.3.4 TVaR of a Lognormal Random Variable
4.3.5 TVaR for Variables with Density c(α)xαg(x)
4.3.6 Algorithm to Evaluate TVaR for a Discrete Distribution
4.3.7 VaR and TVaR for the Case Studies
4.3.8 CTE and WCE: Alternatives to TVaR
4.3.9 TVaR Is Subadditive
4.3.10 TVaR as Solution to an Optimization Problem
4.3.11 Summary: The Different Manifestations of TVaR for a Continuous Variable
4.4 Differentiating Quantiles, VaR, and TVaR
4.5 Learning Objectives
5 Properties of Risk Measures and Advanced Topics
5.1 Probability Scenarios
5.2 Mathematical Properties of Risk Measures
5.2.1 Translation Invariant
5.2.2 Normalized
5.2.3 Monotone
5.2.4 Positive Loading
5.2.5 Monetary Risk Measures
5.2.6 Positive Homogeneous
5.2.7 Lipschitz Continuous
5.2.8 Subadditive
5.2.9 Sublinear
5.2.10 Comonotonic Random Variables
5.2.11 Comonotonic Additive
5.2.12 Independent Additive
5.2.13 Law Invariant
5.2.14 Coherent
5.2.15 Spectral
5.2.16 Convexity Property
5.2.17 Quasi-Convexity
5.2.18 Convex
5.2.19 Acceptance Sets
5.2.20 Compound Risk Measures
5.2.21 Star-Shaped
5.2.22 Risk, Deviation, Regret, and Error
5.3 Risk Preferences
5.3.1 The Preference for a Smaller Loss
5.3.2 The Definition of Increasing Risk
5.3.3 Preferences over Risk Pools
5.3.4 Problems with Utility Theory as a Model of Firm Decision Making
5.3.5 Law Invariance and Second-Order Stochastic Dominance
5.4 The Representation Theorem for Coherent Risk Measures
5.4.1 Handicapping Probability Scenarios
5.4.2 Properties of the Handicapping Function for Coherent Measures
5.4.3 Representation Theorem for Coherent Risk Measures in Detail
5.5 Delbaen’s Differentiation Theorem
5.6 Learning Objectives
5.A Lloyd’s Realistic Disaster Scenarios
5.B Convergence Assumptions for Random Variables
6 Risk Measures in Practice
6.1 Selecting a Risk Measure Using the Characterization Method
6.2 Risk Measures and Risk Margins
6.3 Assessing Tail Risk in a Univariate Distribution
6.4 The Intended Purpose: Applications of Risk Measures
6.4.1 Pricing Risk Measures
6.4.2 Capital Risk Measures
6.5 Compendium of Risk Measures
6.6 Learning Objectives
7 Guide to the Practice Chapters
8 Classical Portfolio Pricing Theory
8.1 Insurance Demand, Supply, and Contracts
8.1.1 Demand
8.1.2 Supply
8.1.3 Insurance Contracts
8.2 Insurer Risk Capital
8.2.1 Assets and Liabilities
8.2.2 Insurer Insolvency, Default, and Priority Rules
8.2.3 Capital vs. Equity
8.2.4 Types of Insurer Capital
8.2.5 Why Insurer Equity Capital Is Expensive
8.2.6 Estimated Cost of Insurer Equity Capital
8.2.7 Weighted Average Cost of Capital
8.2.8 Optimal Capital Structure
8.3 Accounting Valuation Standards
8.3.1 Default Risk, Accounting Value, Economic Value, and Cash Flow
8.3.2 Regulatory Capital and Accounting Frameworks
8.3.3 Taxes
8.4 Actuarial Premium Calculation Principles and Classical Risk Theory
8.4.1 Actuarial Premium Calculation Principles
8.4.2 Classical Risk Theory and the Pollaczeck-Khinchine Formula
8.4.3 Premium Calculation from the Top Down
8.5 Investment Income in Pricing. 8.5.1 US Actuarial Practice
8.5.2 Investment Income in Ratemaking
8.5.3 Timing, Timing Risk, and Amount Risk
8.5.4 The Ferrari Decomposition of Operating Returns
8.6 Financial Valuation and Perfect Market Models
8.7 The Discounted Cash Flow Model
8.7.1 Defining a Fair Rate of Return on Underwriting
8.7.2 Discounted Cash Flow Pricing Formula
8.7.3 The Internal Rate of Return Model
8.7.4 Portfolio Constant Cost of Capital Pricing
8.7.5 Concluding Observations on DCF and IRR Models
8.8 Insurance Option Pricing Models
8.8.1 Development of Insurance Option Pricing Models
8.8.2 Brownian Motion
8.8.3 Stochastic Calculus and Itô’s Lemma
8.8.4 Brownian Motion with Drift
8.8.5 Geometric Brownian Motion
8.8.6 Unique Prices and Risk Adjustments
8.8.7 The Black-Scholes Model
8.8.8 Actuarial vs. Black-Scholes Option Pricing
8.8.9 The Insurance Option Pricing Model
8.8.10 The Historical Importance of Option Pricing Models
8.9 Insurance Market Imperfections
8.9.1 Transaction Costs
8.9.2 Frictional Costs of Capital
8.9.3 Market Frictions
8.9.4 Where We Go from Here
8.10 Learning Objectives
8.A Short- and Long-Duration Contracts
8.A.1 Short-Duration Contracts
8.A.2 Long-Duration Contracts
8.B The Equivalence Principle
9 Classical Portfolio Pricing Practice
9.1 Stand-Alone Classical PCPs
9.1.1 Tame Case Study
9.1.2 Cat/Non-Cat Case Study
9.1.3 Hu/SCS Case Study
9.2 Portfolio CCoC Pricing
9.3 Applications of Classical Risk Theory. 9.3.1 The Pollaczeck-Khinchine Formula
9.3.2 Market Scale and Viability
9.4 Option Pricing Examples
9.5 Learning Objectives
10 Modern Portfolio Pricing Theory
10.1 Classical vs. Modern Pricing and Layer Pricing
10.2 Pricing with Varying Assets
10.3 Pricing by Layer and the Layer Premium Density
10.4 The Layer Premium Density as a Distortion Function
10.5 From Distortion Functions to the Insurance Market
10.5.1 Insurance Market Statistics by Layer
10.5.2 Insurance Market Statistics in Total
10.6 Concave Distortion Functions
10.7 Spectral Risk Measures
10.7.1 SRMs and Adjusted Probabilities, Differentiable g
10.7.2 SRMs and Adjusted Probabilities, General g
10.8 Properties of an SRM and Its Associated Distortion Function
10.9 Six Representations of Spectral Risk Measures
10.10 Simulation Interpretation of Distortion Functions
10.11 Learning Objectives
10.A Technical Details. 10.A.1 Proof of Eq. (10.39)
10.A.2 Proof of Theorem 3
11 Modern Portfolio Pricing Practice
11.1 Applying SRMs to Discrete Random Variables. 11.1.1 Algorithm to Evaluate an SRM on a Discrete Random Variable
11.1.2 Application to the Simple Discrete Example
11.2 Building-Block Distortions and SRMs
11.2.1 Tail Value at Risk
11.2.2 Bi-TVaR
11.2.3 The Distortion Envelope
11.2.4 Piecewise Linear Distortion
11.3 Parametric Families of Distortions
11.3.1 Proportional Hazard
11.3.2 Dual Moment
11.3.3 Wang Transform
11.3.4 Linear Yield
11.3.5 Leverage Equivalent Pricing
11.3.6 The Beta Transform
11.3.7 Altering g
11.4 SRM Pricing
11.4.1 Distortion Function Properties
11.4.2 Pricing by Case Study
11.5 Selecting a Distortion
11.6 Fitting Distortions to Cat Bond Data
11.6.1 Parametric Regression
11.6.2 Bagged Convex Envelopes
11.7 Resolving an Apparent Pricing Paradox
11.7.1 Stand-Alone Resolution
11.7.2 Portfolio Resolution
11.8 Learning Objectives
12 Classical Price Allocation Theory
12.1 The Allocation of Portfolio Constant CoC Pricing
12.2 Allocation of Non-Additive Functionals
12.2.1 Expected Value
12.2.2 Proportional Allocation
12.2.3 Haircut Allocation
12.2.4 Equal Risk Allocations
12.2.5 Marginal Business Unit
12.2.6 Marginal Business Euler Gradient Allocation
12.2.7 Game Theory and the Aumann-Shapley Allocation
12.2.8 Co-Measure Allocations
12.2.9 Exogenous Allocations
12.2.10 The Pricing Implied by a Capital Allocation
12.2.11 Selecting an Allocation Method
12.3 Loss Payments in Default
12.4 The Historical Development of Insurance Pricing Models
12.4.1 Perfect Complete, Model A
12.4.2 Complete, Model B
12.4.3 Limited Liability Complete, Model C
12.4.4 Frictional Cost, Model D
12.4.5 Realistic, Model E
12.4.6 Model Summary
12.5 Learning Objectives
13 Classical Price Allocation Practice
13.1 Allocated CCoC Pricing. 13.1.1 Description
13.1.2 Application
13.1.3 Critique
13.2 Allocation of Classical PCP Pricing
13.3 Learning Objectives
14 Modern Price Allocation Theory
14.1 The Natural Allocation of a Coherent Risk Measure
14.1.1 Lessons from Classical Allocations
14.1.2 Definition of the Natural Allocation
14.1.3 The Linear Natural Allocation
14.1.4 The Lifted Natural Allocation
14.1.5 Constructing the Linear Contact Function from a Distortion
14.2 Computing the Natural Allocations
14.2.1 Algorithm to Compute the Linear Natural Allocation
14.2.2 Algorithm to Compute the Lifted Natural Allocation
14.2.3 Dependence on Assumptions
14.3 A Closer Look at Unit Funding
14.3.1 Conditional Expected Loss: κ
14.3.2 Expected Loss by Asset Level
14.3.3 Premium by Asset Level
14.3.4 The Case with No Default
14.3.5 Summary of Allocation Formulas
14.3.6 Properties of Margin by Unit
14.3.7 Alpha, Beta, and Kappa as Portfolio Diagnostics
14.3.8 The Natural Allocation of Capital
14.3.9 The Percentile Layer of Capital Approach
14.4 An Axiomatic Approach to Allocation
14.4.1 Additive
14.4.2 No-Undercut
14.4.3 Riskless Allocation
14.4.4 Translation Invariant
14.4.5 Monotone
14.4.6 Symmetry
14.4.7 Linear
14.4.8 Decomposable
14.4.9 Continuous
14.4.10 Continuous in Direction
14.4.11 Law Invariant Allocation
14.4.12 Positive Loading
14.5 Axiomatic Characterizations of Allocations
14.5.1 The Shapley Value
14.5.2 The Aumann-Shapley Value
14.5.3 Kalkbrener’s Decomposable and No-Undercut Allocation
14.5.4 Kalkbrener’s Coherent Allocation
14.5.5 Cherny and Orlov’s Linear Natural Allocation
14.5.6 Selecting an Allocation Method, Revisited
14.6 Learning Objectives
15 Modern Price Allocation Practice
15.1 Applying the Natural Allocations to Discrete Random Variables
15.1.1 Algorithm to Compute the Linear Natural Allocation for Discrete Random Variables
15.1.2 Application to the Adjusted Simple Discrete Example
15.1.3 Computing the Natural Allocation Set
15.1.4 Computing the Lifted Natural Allocation
15.2 Unit Funding Analysis
15.2.1 Computing κ
15.2.2 Computing α
15.2.3 Computing β
15.2.4 Computing Margin
15.2.5 Computing Capital and CoC
15.2.6 Computing the Lifted and Linear Natural Allocations
15.2.7 Summary of Formulas
15.3 Bodoff’s Percentile Layer of Capital Method
15.4 Case Study Exhibits. 15.4.1 Visualizing Risk for Case Studies
15.4.2 Applying the Natural Allocation to the Case Studies
15.4.3 Visualizing Spectral Risk Measures
15.4.4 Percentile Layer of Capital Examples
15.5 Learning Objectives
16 Asset Risk
16.1 Background
16.2 Adding Asset Risk to Ins Co
16.3 Learning Objectives
17 Reserves
17.1 Time Periods and Notation
17.2 Liability for Ultimate Losses
17.2.1 The Single Stand Alone Accident Year
17.2.2 The Steady State Portfolio
17.2.3 Problems in Steady State and the Runoff Decision
17.3 The Solvency II Risk Margin
17.4 Learning Objectives
18 Going Concern Franchise Value
18.1 Optimal Dividends
18.2 The Firm Life Annuity
18.3 Learning Objectives
19 Reinsurance Optimization
19.1 Background
19.2 Evaluating Ceded Reinsurance
19.3 Learning Objectives
20 Portfolio Optimization
20.1 Strategic Framework
20.2 Market Regulation
20.3 Dynamic Capital Allocation and Marginal Cost
20.4 Marginal Cost and Marginal Revenue
20.5 Performance Management and Regulatory Rigidities
20.6 Practical Implications
20.7 Learning Objectives
Appendix A Background Material. A.1 Interest Rate, Discount Rate, and Discount Factor
A.2 Actuarial vs. Accounting Sign Conventions
A.3 Probability Theory
A.4 Additional Mathematical Terminology
Appendix B Notation
References
Index
WILEY END USER LICENSE AGREEMENT
Отрывок из книги
Stephen J. Mildenhall and John A. Major
The book came about through a confluence of supporting factors. We had worked independently for many years on the problem of defining the value of risk management and risk transfer (especially in the context of property catastrophe risk) and “escaping the efficient frontier.” Don Mango brought us together to work with him and Jesse Nickerson to present a multipart tutorial on spectral risk measures at the Casualty Actuarial Society Spring 2018 meeting. The tutorial was so successful that we felt it deserved a wider audience and set about developing a monograph: “Spectral Risk Measures for the Working Actuary.” As we proceeded to refine our thinking and presentation, we realized there was so much more to be explained. Three and a half years and 1200 git commits later, we had this book.
.....
(3.4)
(3.5)
.....