Industrial Data Analytics for Diagnosis and Prognosis
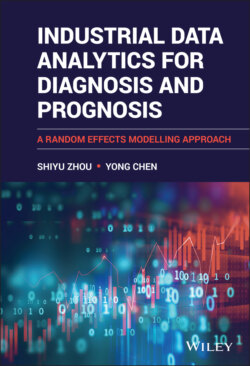
Реклама. ООО «ЛитРес», ИНН: 7719571260.
Оглавление
Yong Chen. Industrial Data Analytics for Diagnosis and Prognosis
Industrial Data Analytics for Diagnosis and Prognosis. A Random Effects Modelling Approach
Contents
Guide
Pages
Preface
Acknowledgments
Acronyms
Table of Notation
1 Introduction. 1.1 Background and Motivation
1.2 Scope and Organization of the Book
1.3 How to Use This Book
Bibliographic Notes
2 Introduction to Data Visualization and Characterization
2.1 Data Visualization
2.1.1 Distribution Plots for a Single Variable
Distribution of A Categorical Variable – Bar Chart
Distribution of Numerical Variables – Histogram and Box Plot
2.1.2 Plots for Relationship Between Two Variables
Relationship Between Two Numerical Variables – Scatter Plot
Relationship Between A Numerical Variable and A Categorical Variable – Side-by-Side Box Plot
Relationship Between Two Categorical Variables – Mosaic Plot
2.1.3 Plots for More than Two Variables
Color Coded Scatter Plot
Scatter Plot Matrix and Heatmap
2.2 Summary Statistics
2.2.1 Sample Mean, Variance, and Covariance. Sample Mean – Measure of Location
Sample Variance – Measure of Spread
Sample Covariance and Correlation – Measure of Linear Association Between Two Variables
2.2.2 Sample Mean Vector and Sample Covariance Matrix
2.2.3 Linear Combination of Variables
Bibliographic Notes
Exercises
3 Random Vectors and the Multivariate Normal Distribution
3.1 Random Vectors
3.2 Density Function and Properties of Multivariate Normal Distribution
Properties of the Multivariate Normal Distribution
3.3 Maximum Likelihood Estimation for Multivariate Normal Distributions
3.4 Hypothesis Testing on Mean Vectors
3.5 Bayesian Inference for Normal Distribution
Bibliographic Notes
Exercises
4 Explaining Covariance Structure: Principal Components. 4.1 Introduction to Principal Component Analysis
4.1.1 Principal Components for More Than Two Variables
4.1.2 PCA with Data Normalization
4.1.3 Visualization of Principal Components
4.1.4 Number of Principal Components to Retain
4.2 Mathematical Formulation of Principal Components
4.2.1 Proportion of Variance Explained
4.2.2 Principal Components Obtained from the Correlation Matrix
4.3 Geometric Interpretation of Principal Components. 4.3.1 Interpretation Based on Rotation
4.3.2 Interpretation Based on Low-Dimensional Approximation
Bibliographic Notes
Exercises
5 Linear Model for Numerical and Categorical Response Variables
5.1 Numerical Response – Linear Regression Models
5.1.1 General Formulation of Linear Regression Model
5.1.2 Significance and Interpretation of Regression Coefficients
5.1.3 Other Types of Predictors in Linear Models
Categorical Predictors
Interactions and Nonlinear Transformation of Variables
5.2 Estimation and Inferences of Model Parameters for Linear Regression
5.2.1 Least Squares Estimation
5.2.2 Maximum Likelihood Estimation
5.2.3 Variable Selection in Linear Regression
5.2.4 Hypothesis Testing
5.3 Categorical Response – Logistic Regression Model
5.3.1 General Formulation of Logistic Regression Model
5.3.2 Significance and Interpretation of Model Coefficients
5.3.3 Maximum Likelihood Estimation for Logistic Regression
Bibliographic Notes
Exercises
6 Linear Mixed Effects Model
6.1 Model Structure
6.2 Parameter Estimation for LME Model. 6.2.1 Maximum Likelihood Estimation Method
6.2.2 Distribution-Free Estimation Methods
6.3 Hypothesis Testing
6.3.1 Testing for Fixed Effects
6.3.2 Testing for Variance–Covariance Parameters
Bibliographic Notes
Exercises
7 Diagnosis of Variation Source Using PCA
7.1 Linking Variation Sources to PCA
7.2 Diagnosis of Single Variation Source
7.3 Diagnosis of Multiple Variation Sources
7.4 Data Driven Method for Diagnosing Variation Sources
Bibliographic Notes
Exercises
8 Diagnosis of Variation Sources Through Random Effects Estimation
8.1 Estimation of Variance Components
8.2 Properties of Variation Source Estimators
8.3 Performance Comparison of Variance Component Estimators
Bibliographic Notes
Exercises
9 Analysis of System Diagnosability
9.1 Diagnosability of Linear Mixed Effects Model
9.2 Minimal Diagnosable Class
9.3 Measurement System Evaluation Based on System Diagnosability
Bibliographic Notes
Exercises
Appendix
10 Prognosis Through Mixed Effects Models for Longitudinal Data
10.1 Mixed Effects Model for Longitudinal Data
10.2 Random Effects Estimation and Prediction for an Individual Unit
10.3 Estimation of Time-to-Failure Distribution
10.4 Mixed Effects Model with Mixture Prior Distribution
10.4.1 Mixture Distribution
10.4.2 Mixed Effects Model with Mixture Prior for Longitudinal Data
10.5 Recursive Estimation of Random Effects Using Kalman Filter
10.5.1 Introduction to the Kalman Filter
10.5.2 Random Effects Estimation Using the Kalman Filter
Biographical Notes
Exercises
Appendix
11 Prognosis Using Gaussian Process Model
11.1 Introduction to Gaussian Process Model
11.2 GP Parameter Estimation and GP Based Prediction
11.3 Pairwise Gaussian Process Model
11.3.1 Introduction to Multi-output Gaussian Process
11.3.2 Pairwise GP Modeling Through Convolution Process
11.4 Multiple Output Gaussian Process for Multiple Signals. 11.4.1 Model Structure
11.4.2 Model Parameter Estimation and Prediction
11.4.3 Time-to-Failure Distribution Based on GP Predictions
Bibliographical Notes
Exercises
12 Prognosis Through Mixed Effects Models for Time-to-Event Data
12.1 Models for Time-to-Event Data Without Covariates
12.1.1 Parametric Models for Time-to-Event Data
12.1.2 Non-parametric Models for Time-to-Event Data
12.2 Survival Regression Models
12.2.1 Cox PH Model with Fixed Covariates
12.2.2 Cox PH Model with Time Varying Covariates
12.2.3 Assessing Goodness of Fit
12.3 Joint Modeling of Time-to-Event Data and Longitudinal Data
12.3.1 Structure of Joint Model and Parameter Estimation
12.3.2 Online Event Prediction for a New Unit
12.4 Cox PH Model with Frailty Term for Recurrent Events
Bibliographical Notes
Exercises
Appendix
Appendix Basics of Vectors, Matrices, and Linear Vector Space. A.1 Vectors and Linear Vector Space
A.2 Matrix and Its Operations
References
Index
WILEY END USER LICENSE AGREEMENT
Отрывок из книги
Shiyu Zhou
University of Wisconsin – Madison
.....
Data visualization is an effective and intuitive representation of the qualitative features of the data. Key characteristics of data can also be quantitatively summarized by numerical statistics. This section introduces common summary statistics for univariate and multivariate data.
A sample mean or sample average provides a measure of location, or central tendency, of a variable in a data set. Consider a univariate data set, which is a data set with a single variable, that consists of a random sample of n observations x1, x2,…, xn. The sample mean is simply the ordinary arithmetic average
.....