Sampling and Estimation from Finite Populations
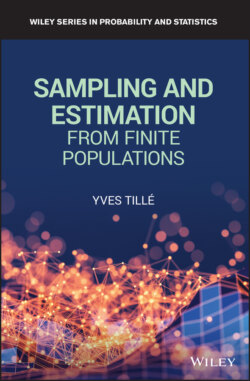
Реклама. ООО «ЛитРес», ИНН: 7719571260.
Оглавление
Yves Tille. Sampling and Estimation from Finite Populations
Table of Contents
List of Tables
List of Illustrations
Guide
Pages
WILEY SERIES IN PROBABILITY AND STATISTICS
Sampling and Estimation from Finite Populations
Copyright
List of Figures
List of Tables
List of Algorithms
Preface
Preface to the First French Edition
Table of Notations
Chapter 1 A History of Ideas in Survey Sampling Theory. 1.1 Introduction
1.2 Enumerative Statistics During the 19th Century
1.3 Controversy on the use of Partial Data
1.4 Development of a Survey Sampling Theory
1.5 The US Elections of 1936
1.6 The Statistical Theory of Survey Sampling
1.7 Modeling the Population
1.8 Attempt to a Synthesis
1.9 Auxiliary Information
1.10 Recent References and Development
Notes
Chapter 2 Population, Sample, and Estimation. 2.1 Population
2.2 Sample
Definition 2.1
Definition 2.2
Definition 2.3
2.3 Inclusion Probabilities
Result 2.1
Proof:
Result 2.2
Proof:
Example 2.1
2.4 Parameter Estimation
Definition 2.4
Definition 2.5
Definition 2.6
Result 2.3
Proof:
2.5 Estimation of a Total
Result 2.4
Proof:
2.6 Estimation of a Mean
Definition 2.7
2.7 Variance of the Total Estimator
Result 2.5
Proof:
Result 2.6
Proof:
Result 2.7
Proof:
Definition 2.8
2.8 Sampling with Replacement
Exercises
Chapter 3 Simple and Systematic Designs
3.1 Simple Random Sampling without Replacement with Fixed Sample Size. 3.1.1 Sampling Design and Inclusion Probabilities
Definition 3.1
3.1.2 The Expansion Estimator and its Variance
Result 3.1
Proof:
Result 3.2
Proof:
Result 3.3
Proof:
3.1.3 Comment on the Variance–Covariance Matrix
3.2 Bernoulli Sampling. 3.2.1 Sampling Design and Inclusion Probabilities
Definition 3.2
3.2.2 Estimation
3.3 Simple Random Sampling with Replacement
Result 3.4
Proof:
Result 3.5
Proof:
3.4 Comparison of the Designs with and Without Replacement
3.5 Sampling with Replacement and Retaining Distinct Units. 3.5.1 Sample Size and Sampling Design
Result 3.6
Proof:
Result 3.7
Proof:
3.5.2 Inclusion Probabilities and Estimation
Result 3.8
Proof:
Result 3.9
Proof:
3.5.3 Comparison of the Estimators
Result 3.10
Proof:
3.6 Inverse Sampling with Replacement
Example 3.1
3.7 Estimation of Other Functions of Interest. 3.7.1 Estimation of a Count or a Proportion
3.7.2 Estimation of a Ratio
3.8 Determination of the Sample Size
3.9 Implementation of Simple Random Sampling Designs. 3.9.1 Objectives and Principles
3.9.2 Bernoulli Sampling
3.9.3 Successive Drawing of the Units
3.9.4 Random Sorting Method
Result 3.11 (Sunter, 1977) [p. 263]
Proof:
3.9.5 Selection–Rejection Method
Result 3.12
Proof:
3.9.6 The Reservoir Method
Result 3.13 (McLeod & Bellhouse, 1983)
Proof:
3.9.7 Implementation of Simple Random Sampling with Replacement
3.10 Systematic Sampling with Equal Probabilities
3.11 Entropy for Simple and Systematic Designs
3.11.1 Bernoulli Designs and Entropy
Result 3.14
Proof:
Result 3.15
Proof:
3.11.2 Entropy and Simple Random Sampling
Result 3.16
Proof:
3.11.3 General Remarks
Exercises
Chapter 4 Stratification
4.1 Population and Strata
4.2 Sample, Inclusion Probabilities, and Estimation
Definition 4.1
4.3 Simple Stratified Designs
4.4 Stratified Design with Proportional Allocation
Definition 4.2
4.5 Optimal Stratified Design for the Total
Result 4.1
Proof:
Example 4.1
4.6 Notes About Optimality in Stratification
4.7 Power Allocation
4.8 Optimality and Cost
4.9 Smallest Sample Size
4.10 Construction of the Strata. 4.10.1 General Comments
4.10.2 Dividing a Quantitative Variable in Strata
4.11 Stratification Under Many Objectives
Exercises
Chapter 5 Sampling with Unequal Probabilities
5.1 Auxiliary Variables and Inclusion Probabilities
5.2 Calculation of the Inclusion Probabilities
Example 5.1
5.3 General Remarks
5.4 Sampling with Replacement with Unequal Inclusion Probabilities
Result 5.1
Proof:
Result 5.2
Proof:
5.5 Nonvalidity of the Generalization of the Successive Drawing without Replacement
5.6 Systematic Sampling with Unequal Probabilities
Example 5.2
5.7 Deville's Systematic Sampling
5.8 Poisson Sampling
Result 5.3
Proof:
5.9 Maximum Entropy Design
Result 5.4
Proof:
Result 5.5
Proof:
Result 5.6 (Deville, 2000b)
Proof:
5.10 Rao–Sampford Rejective Procedure
Result 5.7
5.11 Order Sampling
5.12 Splitting Method. 5.12.1 General Principles
5.12.2 Minimum Support Design
Definition 5.1
Example 5.3
5.12.3 Decomposition into Simple Random Sampling Designs
Example 5.4
5.12.4 Pivotal Method
5.12.5 Brewer Method
Result 5.8
Proof:
5.13 Choice of Method
Result 5.9
Proof
5.14 Variance Approximation
5.15 Variance Estimation
Exercises
Chapter 6 Balanced Sampling. 6.1 Introduction
6.2 Balanced Sampling: Definition
Definition 6.1
Example 6.1
Example 6.2
Example 6.3
6.3 Balanced Sampling and Linear Programming
Result 6.1
Proof:
6.4 Balanced Sampling by Systematic Sampling
6.5 Methode of Deville, Grosbras, and Roth
6.6 Cube Method. 6.6.1 Representation of a Sampling Design in the form of a Cube
6.6.2 Constraint Subspace
Example 6.4
6.6.3 Representation of the Rounding Problem
Example 6.5
Example 6.6
Definition 6.2
Definition 6.3
Definition 6.4
Result 6.2
Proof:
6.6.4 Principle of the Cube Method
6.6.5 The Flight Phase
6.6.6 Landing Phase by Linear Programming
Definition 6.5
6.6.7 Choice of the Cost Function
Result 6.3
Proof:
6.6.8 Landing Phase by Relaxing Variables
6.6.9 Quality of Balancing
Result 6.4
Proof:
6.6.10 An Example
6.7 Variance Approximation
6.8 Variance Estimation
6.9 Special Cases of Balanced Sampling
6.10 Practical Aspects of Balanced Sampling
Exercise
Chapter 7 Cluster and Two‐stage Sampling
7.1 Cluster Sampling
7.1.1 Notation and Definitions
7.1.2 Cluster Sampling with Equal Probabilities
7.1.3 Sampling Proportional to Size
7.2 Two‐stage Sampling
7.2.1 Population, Primary, and Secondary Units
7.2.2 The Expansion Estimator and its Variance
Result 7.1
Proof:
Result 7.2
Proof:
Result 7.3
Proof:
7.2.3 Sampling with Equal Probability
7.2.4 Self‐weighting Two‐stage Design
7.3 Multi‐stage Designs
7.4 Selecting Primary Units with Replacement
Result 7.4
Proof:
Result 7.5
Proof:
7.5 Two‐phase Designs. 7.5.1 Design and Estimation
Example 7.1
7.5.2 Variance and Variance Estimation
Result 7.6
Proof:
7.6 Intersection of Two Independent Samples
Exercises
Chapter 8 Other Topics on Sampling. 8.1 Spatial Sampling. 8.1.1 The Problem
8.1.2 Generalized Random Tessellation Stratified Sampling
8.1.3 Using the Traveling Salesman Method
8.1.4 The Local Pivotal Method
8.1.5 The Local Cube Method
8.1.6 Measures of Spread
8.2 Coordination in Repeated Surveys. 8.2.1 The Problem
8.2.2 Population, Sample, and Sample Design
Result 8.1
Proof:
8.2.3 Sample Coordination and Response Burden. Definition 8.1
Definition 8.2
8.2.4 Poisson Method with Permanent Random Numbers
8.2.5 Kish and Scott Method for Stratified Samples
8.2.6 The Cotton and Hesse Method
8.2.7 The Rivière Method
8.2.8 The Netherlands Method
8.2.9 The Swiss Method
8.2.10 Coordinating Unequal Probability Designs with Fixed Size
8.2.11 Remarks
8.3 Multiple Survey Frames. 8.3.1 Introduction
Example 8.1
Example 8.2
8.3.2 Calculating Inclusion Probabilities
8.3.3 Using Inclusion Probability Sums
Result 8.2
Proof:
8.3.4 Using a Multiplicity Variable
Result 8.3
Proof:
8.3.5 Using a Weighted Multiplicity Variable
8.3.6 Remarks
8.4 Indirect Sampling. 8.4.1 Introduction
Example 8.3
Example 8.4
Example 8.5
Example 8.6
8.4.2 Adaptive Sampling
8.4.3 Snowball Sampling
8.4.4 Indirect Sampling
8.4.5 The Generalized Weight Sharing Method
Result 8.4
Proof:
8.5 Capture–Recapture
Example 8.7
Chapter 9 Estimation with a Quantitative Auxiliary Variable
9.1 The Problem
Definition 9.1
9.2 Ratio Estimator. 9.2.1 Motivation and Definition
9.2.2 Approximate Bias of the Ratio Estimator
9.2.3 Approximate Variance of the Ratio Estimator
9.2.4 Bias Ratio
9.2.5 Ratio and Stratified Designs
9.3 The Difference Estimator
9.4 Estimation by Regression
9.5 The Optimal Regression Estimator
9.6 Discussion of the Three Estimation Methods
Exercises
Chapter 10 Post‐Stratification and Calibration on Marginal Totals. 10.1 Introduction
10.2 Post‐Stratification. 10.2.1 Notation and Definitions
10.2.2 Post‐Stratified Estimator
10.3 The Post‐Stratified Estimator in Simple Designs. 10.3.1 Estimator
10.3.2 Conditioning in a Simple Design
Result 10.1
Proof:
10.3.3 Properties of the Estimator in a Simple Design
10.4 Estimation by Calibration on Marginal Totals
10.4.1 The Problem
10.4.2 Calibration on Marginal Totals
10.4.3 Calibration and Kullback–Leibler Divergence
10.4.4 Raking Ratio Estimation
10.5 Example
Exercises
Chapter 11 Multiple Regression Estimation. 11.1 Introduction
Definition 11.1
11.2 Multiple Regression Estimator
11.3 Alternative Forms of the Estimator. 11.3.1 Homogeneous Linear Estimator
11.3.2 Projective Form
Result 11.1
Proof:
11.3.3 Cosmetic Form
Result 11.2
Proof:
11.4 Calibration of the Multiple Regression Estimator
11.5 Variance of the Multiple Regression Estimator
11.6 Choice of Weights
11.7 Special Cases
11.7.1 Ratio Estimator
11.7.2 Post‐stratified Estimator
11.7.3 Regression Estimation with a Single Explanatory Variable
11.7.4 Optimal Regression Estimator
11.7.5 Conditional Estimation
Result 11.3
Proof:
11.8 Extension to Regression Estimation
Exercise
Chapter 12 Calibration Estimation. 12.1 Calibrated Methods
12.2 Distances and Calibration Functions. 12.2.1 The Linear Method
12.2.2 The Raking Ratio Method
12.2.3 Pseudo Empirical Likelihood
12.2.4 Reverse Information
12.2.5 The Truncated Linear Method
12.2.6 General Pseudo‐Distance
12.2.7 The Logistic Method
12.2.8 Deville Calibration Function
12.2.9 Roy and Vanheuverzwyn Method
12.3 Solving Calibration Equations
12.3.1 Solving by Newton's Method
12.3.2 Bound Management
12.3.3 Improper Calibration Functions
12.3.4 Existence of a Solution
12.4 Calibrating on Households and Individuals
12.5 Generalized Calibration. 12.5.1 Calibration Equations
12.5.2 Linear Calibration Functions
Remark 12.1
12.6 Calibration in Practice
12.7 An Example
Exercises
Chapter 13 Model‐Based approach. 13.1 Model Approach
13.2 The Model
Result 13.1
Proof:
Result 13.2
Proof:
Result 13.3
Proof:
13.3 Homoscedastic Constant Model
Result 13.4
Proof:
13.4 Heteroscedastic Model 1 Without Intercept
Result 13.5
Proof:
13.5 Heteroscedastic Model 2 Without Intercept
Result 13.6
Proof:
13.6 Univariate Homoscedastic Linear Model
Result 13.7
Proof:
13.7 Stratified Population
13.8 Simplified Versions of the Optimal Estimator
Definition 13.1
Result 13.8
Proof:
Result 13.9
Proof:
13.9 Completed Heteroscedasticity Model
13.10 Discussion
13.11 An Approach that is Both Model‐ and Design‐based
Result 13.10
Proof:
Result 13.11
Proof:
Chapter 14 Estimation of Complex Parameters. 14.1 Estimation of a Function of Totals
Example 14.1
Example 14.2
14.2 Variance Estimation
14.3 Covariance Estimation
14.4 Implicit Function Estimation
Example 14.3
14.5 Cumulative Distribution Function and Quantiles. 14.5.1 Cumulative Distribution Function Estimation
14.5.2 Quantile Estimation: Method 1
Example 14.4
14.5.3 Quantile Estimation: Method 2
Example 14.5
14.5.4 Quantile Estimation: Method 3
Example 14.6
14.5.5 Quantile Estimation: Method 4
14.6 Cumulative Income, Lorenz Curve, and Quintile Share Ratio. 14.6.1 Cumulative Income Estimation
14.6.2 Lorenz Curve Estimation
14.6.3 Quintile Share Ratio Estimation
14.7 Gini Index
Result 14.1
Proof:
Result 14.2
Proof:
14.8 An Example
Chapter 15 Variance Estimation by Linearization. 15.1 Introduction
15.2 Orders of Magnitude in Probability
Definition 15.1
Definition 15.2
Definition 15.3
Definition 15.4
Definition 15.5
Result 15.1
Proof:
Result 15.2
Theorem 15.1
Proof:
Result 15.3
Proof:
Result 15.4
Proof:
Example 15.1
Result 15.5
Proof:
Result 15.6
Proof:
15.3 Asymptotic Hypotheses
Definition 15.6
Definition 15.7
15.3.1 Linearizing a Function of Totals
Result 15.7
Proof:
15.3.2 Variance Estimation
15.4 Linearization of Functions of Interest
15.4.1 Linearization of a Ratio
15.4.2 Linearization of a Ratio Estimator
15.4.3 Linearization of a Geometric Mean
15.4.4 Linearization of a Variance
15.4.5 Linearization of a Covariance
15.4.6 Linearization of a Vector of Regression Coefficients
15.5 Linearization by Steps
15.5.1 Decomposition of Linearization by Steps
Example 15.2
15.5.2 Linearization of a Regression Coefficient
15.5.3 Linearization of a Univariate Regression Estimator
15.5.4 Linearization of a Multiple Regression Estimator
15.6 Linearization of an Implicit Function of Interest. 15.6.1 Estimating Equation and Implicit Function of Interest
Example 15.3
15.6.2 Linearization of a Logistic Regression Coefficient
15.6.3 Linearization of a Calibration Equation Parameter
15.6.4 Linearization of a Calibrated Estimator
15.7 Influence Function Approach. 15.7.1 Function of Interest, Functional
15.7.2 Definition
15.7.3 Linearization of a Total. Result 15.8
15.7.4 Linearization of a Function of Totals
Result 15.9
Proof:
Example 15.4
Example 15.5
Example 15.6
15.7.5 Linearization of Sums and Products. Result 15.10
Proof:
Result 15.11
Proof:
15.7.6 Linearization by Steps
Result 15.12
Proof:
15.7.7 Linearization of a Parameter Defined by an Implicit Function
Result 15.13
Proof:
Example 15.7
15.7.8 Linearization of a Double Sum
Result 15.14
Proof:
Example 15.8
Example 15.9
15.8 Binder's Cookbook Approach
Example 15.10
15.9 Demnati and Rao Approach
Example 15.11
Example 15.12
Result 15.15
Proof:
15.10 Linearization by the Sample Indicator Variables. 15.10.1 The Method
Example 15.13
Example 15.14
Example 15.15
Example 15.16
Example 15.17
15.10.2 Linearization of a Quantile
15.10.3 Linearization of a Calibrated Estimator
Result 15.16
Proof:
15.10.4 Linearization of a Multiple Regression Estimator
15.10.5 Linearization of an Estimator of a Complex Function with Calibrated Weights
Result 15.17
Proof:
15.10.6 Linearization of the Gini Index
15.11 Discussion on Variance Estimation
Exercises
Chapter 16 Treatment of Nonresponse
16.1 Sources of Error
16.2 Coverage Errors
16.3 Different Types of Nonresponse
16.4 Nonresponse Modeling
16.5 Treating Nonresponse by Reweighting. 16.5.1 Nonresponse Coming from a Sample
16.5.2 Modeling the Nonresponse Mechanism
16.5.3 Direct Calibration of Nonresponse
Example 16.1
16.5.4 Reweighting by Generalized Calibration
16.6 Imputation. 16.6.1 General Principles
16.6.2 Imputing From an Existing Value
16.6.3 Imputation by Prediction
16.6.4 Link Between Regression Imputation and Reweighting
Result 16.1
Proof:
Example 16.2
16.6.5 Random Imputation
16.7 Variance Estimation with Nonresponse. 16.7.1 General Principles
16.7.2 Estimation by Direct Calibration
Result 16.2
Proof:
16.7.3 General Case
16.7.4 Variance for Maximum Likelihood Estimation
Result 16.3
Proof:
Result 16.4
Proof:
Example 16.3
16.7.5 Variance for Estimation by Calibration
Result 16.5
Proof:
Result 16.6
Proof:
16.7.6 Variance of an Estimator Imputed by Regression
16.7.7 Other Variance Estimation Techniques
Chapter 17 Summary Solutions to the Exercises
Bibliography
Author Index
Subject Index
WILEY END USER LICENSE AGREEMENT
Отрывок из книги
Established by WALTER A. SHEWHART and SAMUEL S. WILKS
Editors: Noel Cressie, Garrett Fitzmaurice, David Balding, Geert Molenberghs, Geof Givens, Harvey Goldstein, David Scott, Adrian Smith, Ruey Tsay.
.....
the bias of the estimator is
The bias is zero if and only if for all
.....