Optimizations and Programming
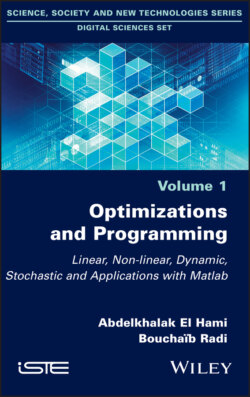
Реклама. ООО «ЛитРес», ИНН: 7719571260.
Оглавление
Abdelkhalak El Hami. Optimizations and Programming
Table of Contents
List of Tables
List of Illustrations
Guide
Pages
Optimizations and Programming. Linear, Nonlinear, Dynamic, Stochastic and Applications with Matlab
Preface
Acknowledgments
1. Linear Programming. 1.1. Introduction
1.2. Definitions
Terminology
1.3. Geometry of the linear program. 1.3.1. Polyhedra
1.3.2. Extreme points and vertices
1.4. Graphical solving of a linear program
1.5. Simplex algorithm
1.5.1. Basic solutions and basic feasible solutions
1.5.2. Simplex tableau
General case
1.5.3. Change of feasible basis
Calculating the new tableau
1.5.4. Existence and uniqueness of an optimal solution
1.6. Initialization of the simplex algorithm
1.6.1. Big M method
1.6.2. Auxiliary program or Phase I
1.6.3. Degeneracy and cycling
1.6.4. Geometric structure of realizable solutions
1.7. Interior-point algorithm
1.8. Duality
1.8.1. Duality theorem
Application
1.9. Relaxation
1.9.1. Lagrangian relaxation
1.10. Postoptimal analysis
1.10.1. Effect of modifying b
1.10.2. Effect of modifying c
Case of a non-basic variable
1.11. Application to an inventory problem
1.11.1. Optimal solution
1.11.2. Sensitivity to variation in stock
1.11.3. Dual problem of the competitor
1.12. Using Matlab
2. Integer Programming. 2.1. Introduction
2.2. Solving methods
2.2.1. Branch-and-bound method
2.2.2. The branch-and-cut method
2.3. Binary programming
2.3.1. Knapsack problem
2.3.2. Investment problem
2.4. Decomposition principle
2.4.1. Benders decomposition
2.5. Using Matlab
3. Dynamic Programming. 3.1. Introduction
3.2. Solving strategy
3.3. Discrete DP
3.3.1. Bellman’s equation and the principle of optimality
3.3.2. Approach of the method
3.3.3. A few examples of DP. 3.3.3.1. Example of a mailman
3.3.4. Solving an LP
3.3.5. Shortest path problem
3.3.6. Knapsack problem
3.3.7. Stock management problem
3.4. Continuous DP
3.4.1. Hamilton–Jacobi equation
3.4.2. Application to a consumption-savings model
3.5. Stochastic DP
3.5.1. Decision-chance process
3.5.2. Solving method
3.5.3. Application to a contract problem
3.5.4. Optimal binary search tree
3.5.4.1. Formulation of the problem
3.5.4.2. Formulation of the solution
3.6. Using Matlab
4. Stochastic Programming. 4.1. Introduction
4.2. Presentation of the problem
4.3. Optimal feedback in an open loop
4.4. Stochastic linear programming
4.4.1. Models with probability thresholds on the constraints
4.5. Stochastic linear programs with recourse
4.5.1. L-shaped method
4.5.2. Multicut L-shaped method
4.5.3. Interior linearization method
4.6. Nonlinear stochastic programming. 4.6.1. Approaches to two-step problems with recourse
4.6.2. Regularized decomposition method
4.6.3. Methods based on the Lagrangian
4.6.4. Frank–Wolfe method for problems with simple recourse
4.6.5. Approximation by sampling average: Monte Carlo method
4.6.6. Stochastic gradient method
4.7. Stochastic dynamic programming
4.7.1. Markov decision process
4.7.2. Scenario tree
4.8. Application to the reliability of mechanical systems
4.8.1. Position and modeling of the reliability problem
4.8.1.1. Deterministic mechanical model
4.8.1.2. Randomness and probabilistic modeling
4.8.1.3. Failure modes of a structure
4.8.1.4. Failure probability of a structure
4.8.1.5. Hasofer and Lind index
4.8.1.6. FORM method
4.9. Using Matlab
5. Combinatorial Optimization. 5.1. Introduction
5.2. Symmetric TSP
5.2.1. Historical overview
5.2.2. Solving methods. 5.2.2.1. Exact methods
5.2.2.2. Approximate methods
5.3. Asymmetric traveling salesman problem
5.3.1. Variants of the ATSP
5.3.2. Mathematical formulations
5.3.2.1. DFJ formulation
5.3.2.2. MTZ formulation
5.3.2.3. Desrochers–Laporte formulation
5.3.3. Methods for solving the ATSP
5.3.3.1. Branch-and-bound method
5.3.3.2. Cutting-plane method
5.3.3.3. Branch-and-cut method
5.4. Vehicle routing problem. 5.4.1. Definition
5.4.2. Fields of application
5.4.3. Parameters of the VRP
5.4.4. Variants of the VRP
5.4.5. Mathematical formulation of the VRP
Formulation
5.4.6. Algorithmic complexity
5.5. Selective routing problem
5.5.1. Problems similar to the VRP
5.5.2. Mathematical formulation
5.6. Using Matlab
6. Unconstrained Nonlinear Programming. 6.1. Introduction
6.2. Mathematical formulation
6.2.1. Existence and uniqueness results
6.3. Optimality conditions
6.4. Quadratic problems
6.4.1. Gradient method with optimal step size
6.4.2. Conjugate gradient method
6.5. Newton’s algorithm
6.6. Methods of descent and linear search. 6.6.1. Presentation of methods of descent
6.6.2. Method of greatest slope
6.6.3. Acceptable step size
6.6.4. Linear search
6.6.5. Newton’s method with linear search
6.7. Quasi-Newton methods
6.7.1. DFP and BFGS methods
6.8. Relaxation method
6.9. Gradient method
6.10. Least squares problem
6.10.1. Gauss–Newton method
6.10.2. Levenberg–Marquardt algorithm
6.10.3. Kalman filter
6.11. Direct search methods
6.11.1. Nelder–Mead algorithm
6.11.2. Torczon method
6.12. Application to an identification problem
6.13. Using Matlab
6.13.1. The fminsearch function
6.13.2. The fminunc function
6.13.3. Relaxation method
7. Constrained Nonlinear Optimization. 7.1. Introduction
7.2. Mathematical formulation
7.3. Lagrange multipliers
7.4. Optimization with inequality constraints
7.4.1. First-order conditions of optimality. 7.4.1.1. Lagrange conditions
7.4.1.2. Karush–Kuhn–Tucker conditions
7.4.2. Presentation of saddle points
7.4.3. Saddle point and optimization
7.4.4. Convex case
7.5. Constrained minimization algorithms
7.5.1. Relaxation method
7.5.2. Projection method
7.5.3. Exterior penalty method
7.5.4. Uzawa’s algorithm
7.6. Newton algorithms: SQP method
7.6.1. Equality constraints
7.6.2. Inequality constraints
7.7. Application to structure optimization
7.8. Using Matlab
7.8.1. The fmincon function
7.8.2. The fminbnd function
7.8.3. Penalty method
Appendix 1. Reminders from Linear Algebra
A1.1. Vector space. A1.1.1. General definitions
A1.1.2. Free families, generating families and bases
A1.2. Linear mappings
A1.3. Matrices
A1.3.1. Operations on matrices
Properties
A1.3.2. Change of basis matrices
A1.3.3. Matrix notation
A1.4. Determinants
Properties
Properties
A1.5. Scalar product
A1.6. Vector norm
Appendix 2. Reminders about functions from ℝn into ℝ
A2.1. Differentiability
A2.2. Convexity
A2.3. Quadratic function
Appendix 3. Optimization Toolbox. A3.1. Introduction
A3.2. Various functions
A3.3. Matlab’s optimization application
Appendix 4. Software. A4.1. Autonomous and multipurpose optimization software
A4.2. Packages for specific classes of problems
A4.3. Optimization software for design
A4.4. Solvers for stochastic optimization
References
Index
WILEY END USER LICENSE AGREEMENT
Отрывок из книги
Digital Sciences Set
.....
More recently, Bland [BLA 77] suggested modifying the rules for choosing the change of basis. Bland’s rule proceeds as follows:
Earlier, when we solved linear programs graphically, the optimal solutions were on the boundary of the convex set of realizable solutions. If there was a unique optimal solution, it was an extreme point.
.....