Fault Diagnosis, Prognosis, and Reliability for Electrical Machines and Drives
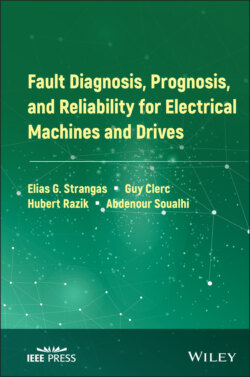
Реклама. ООО «ЛитРес», ИНН: 7719571260.
Оглавление
Abdenour Soualhi. Fault Diagnosis, Prognosis, and Reliability for Electrical Machines and Drives
Fault Diagnosis, Prognosis, and Reliability for Electrical Machines and Drives
Contents
List of Illustrations
List of Tables
Guide
Pages
Contributors
Acknowledgments
Acronyms
Introduction
1 Basic Methods and Tools. 1.1 General Approach
1.2 Feature Extraction: Signal and Preconditioning. 1.2.1 Raw Signals: What Kind of Signals and Sensors?
1.2.1.1 Current Sensors
1.2.1.2 Vibration Measurement and Accelerometers
1.2.1.3 Temperature Sensors
1.2.1.4 Field Sensors
1.2.1.5 Acoustic Sensors
1.2.1.6 Other Sensors
1.2.2 Preconditioning. 1.2.2.1 Signal Features in the Time Domain
1.2.2.2 Symmetric Component, Park Component
1.2.2.3 Symmetric Component, Park Component
1.2.2.4 Signal Features in the Frequency Domain
(2) Fast Fourier transform (recursive Fourier transform)
1.2.2.5 Wavelet Analysis
1.2.2.6 Instantaneous Amplitude and Frequency (1) Instantaneous amplitude
(2) Instantaneous frequency
1.2.2.7 Bilinear Time-frequency Distributions or Quadratic Time-frequency Distributions: Cohen’s Class
1.2.2.7.a Uncertainty principle of Heisenberg
1.2.2.7.b General representation
1.2.2.7.c Properties
1.2.2.7.d Different representations
1.2.2.8 Statistic Features
1.2.2.9 Cyclostationarity (1) General description
(2) nth-order strict cyclostationarity
1.2.3 Model Approach
1.2.3.1 Kalman Observer
1.2.3.2 Extended Observer
1.2.3.3 Unscented Kalman Filter
1.2.4 Parity Space
1.3 Feature Reduction, Principal Component Analysis
1.3.1 Principal Component Analysis: A Space Reduction and an Unsupervised Classification
1.3.2 Intercorrelation
1.3.2.1 Pearson Coefficient “r”
1.3.2.2 Spearman Coefficient “ρ”
1.3.3 Information Content: Shannon Entropy
1.3.4 Pattern Sizing Reduction for a Supervised Classification
1.3.4.1 Selection Criteria
1.3.4.2 Sequential Backward Feature Selection and Sequential Forward Feature Selection
1.3.5 Pattern Sizing Reduction for an Unsupervised Classification: Laplacian Score
1.3.6 Choice of the Number of Classes for an Unsupervised Classification
1.3.6.1 Choice of the Number of Classes with a PCA
1.3.6.2 General Case
1.3.7 Other Quality Criteria of a Classification
1.3.7.1 R2 index
1.3.7.2 Calinski–Harabasz Index
1.3.7.3 Davies–Bouldin Index
1.3.7.4 Silhouette Index
1.3.7.5 Dunn Index
1.4 Classification Methods. 1.4.1 Generalities
1.4.1.1 Supervised and Unsupervised Clustering
1.4.1.2 Measuring the Similarity: Different Distances
1.4.2 Supervised Clustering
1.4.2.1 k Nearest Neighbors
1.4.2.2 Support Vector Machine
1.4.2.3 Recurrent Neural Network
1.4.3 Unsupervised Clustering
1.4.3.1 Hierarchical Classification
(1) Linkage criterion
1.4.3.2 K-means and Centroid Clustering
1.4.3.3 Self-organizing Map
1.5 Prognosis Methods. 1.5.1 Prognosis Process
1.5.2 Time Series Extrapolation Methods
(0)1 Definition
(2) Estimation of the trend ([106])
1.5.3 Bayesian Inference
1.5.4 Markov Chain
1.5.5 Hidden Markov Models
1.5.6 Rainflow
1.5.6.1 Hidden Semi-Markov Models
References
2 Applications and Specifics. 2.1 General Presentation of Motor Drives
2.2 Electrical Machines
2.2.1 Basics
2.2.2 Magnetic Steel and Magnets
2.2.3 Windings and Insulation
2.3 Machine Models, Operation, and Control. 2.3.1 Three-phase Windings
2.3.2 Induction Machines
2.3.2.1 Induction Machine Rotor Field Orientation
2.3.2.2 Direct Torque Control
2.3.3 Permanent Magnet AC Machines
2.4 Faults in Electrical Machines
2.4.1 Operational Variables and Measurements
2.4.2 Supervision, Detection, and Fault Classification
2.4.3 Bearings
2.4.4 Insulation
2.5 Open and Short Faults, Eccentricity, Broken Magnets and Rotor Bars
2.5.1 Induction Machines
2.5.1.1 Stator Fault Diagnosis
2.5.1.2 Eccentricity
2.5.1.3 Multi-fault Diagnosis with Stray Flux and Flux Sensor
2.5.1.4 Open Faults in Windings and Inverter
2.5.1.5 Broken Rotor Bars
2.5.2 Permanent Magnet AC Machines. 2.5.2.1 Demagnetization of Permanent Magnets
2.5.2.2 Open and Short Circuit
2.5.3 Sensor Faults
2.5.4 Fault Mitigation and Management
2.6 Power Electronics and Systems
2.6.1 A Brief Description of Power Electronics in AC drives
2.6.2 A Brief Description of Static Switches
2.6.2.1 Mosfet
2.6.2.2 Igbt
2.6.2.3 Si and SiC Technology
2.6.2.4 Thermal Behavior
2.6.3 A Brief Description of Capacitors. 2.6.3.1 General Description
2.6.3.2 Different Kinds of Capacitors. 2.6.3.2.a Non-polarized Capacitors (1) Air
(2) Ceramic
(3) Plastic
2.6.3.2.b Polarized Capacitors
(4) Tantalum capacitors
2.6.4 Device Faults and Their Manifestation
2.6.4.1 Basic Notion
2.6.4.2 On Chip Failures
2.6.4.3 Packaging and Chip Environment Failures
2.6.5 Capacitor Failure Modes
2.6.5.1 Failure by Degradation
2.6.5.2 Catastrophic Failure
2.6.6 Diagnosis and Prognosis Techniques for Power Devices. 2.6.6.1 Introduction
2.6.6.2 Failure Modes Indicators and TSEP for Power Electronic Devices
2.6.6.3 Diagnosis of Failure Modes
2.6.6.3.a Diagnosis based on the direct analysis of the current (1) Park’s transformation
(2) Slope of current
(3) Average current
(4) Fuzzy technique
2.6.6.3.b Diagnosis based on the direct or indirect analysis of junction temperature
(5) Diagnosis based on TSEPs (see 2.6.6.2)
(6) Diagnosis based on a thermal model
2.6.6.3.c Diagnosis based on signal processing
(7) Frequency analysis
(8) Instantaneous frequency method
(9) Wavelet analysis
2.6.6.3.d Diagnosis based on clustering
2.6.6.3.e Diagnosis based on neural network
2.6.6.3.f Synthesis
2.6.6.4 Prognosis of Failure Modes
2.6.6.4.a Prognosis based on failure mechanism and statistical data
2.6.6.4.b Prognosis based on Failure Precursors
(1) Conventional numerical techniques: Regressors, Kalman filters, Particle filter, etc
(2) Machine learning techniques
(3) Fuzzy methods
2.6.7 Diagnosis and Prognosis Techniques for Capacitors. 2.6.7.1 Fault Diagnosis Techniques
2.6.7.2 Methods for Predicting Electrolytic Capacitor Failures
Bibliography
3 Fault Diagnosis and Prognosis for Reliability Enhancement. 3.1 Introduction
3.2 Fundamentals
3.2.1 The Pattern of Failures with Time for Non-Repairable Items
3.2.2 Distribution Functions
3.2.3 Confidence in Reliability and Prognosis
3.3 Component Reliability
3.4 Reliability of Subsystems and Systems. 3.4.1 Analysis Tools
3.5 Lifetime, Reliability Prediction
3.6 Fault Management and Mitigation
3.7 Design and Manufacturing
3.8 Applications and Case Studies
3.9 Scheduled Maintenance, Condition-Based Maintenance and Prognosis-Enhanced Reliability
3.9.1 Reliability and Costs
3.10 Conclusions
Bibliography
Index
WILEY END USER LICENSE AGREEMENT
Отрывок из книги
Elias G. Strangas
Michigan State UniversityEast Lansing, Michigan
.....
University of LyonVilleurbanne, France
Abdenour Soualhi
.....