Medical Statistics
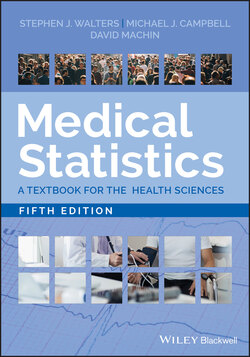
Реклама. ООО «ЛитРес», ИНН: 7719571260.
Оглавление
David Machin. Medical Statistics
Table of Contents
List of Tables
List of Illustrations
Guide
Pages
Medical Statistics. A Textbook for the Health Sciences
Preface
1 Uses and Abuses of Medical Statistics
Summary
1.1 Introduction
1.2 Why Use Statistics?
1.3 Statistics is About Common Sense and Good Design
Example from the Literature – Drinking Coffee and Cancer (IARC 2018)
1.4 How a Statistician Can Help
Sample Size and Power Considerations
Questionnaires
Choice of Sample and of Control Subjects
Design of Study
Laboratory Experiments
Displaying Data
Choice of Summary Statistics and Statistical Analysis
Medical Statistics and Data Science
2 Displaying and Summarising Data
Summary
2.1 Types of Data
Example from the Literature – Salicylic Acid Plasters for Treatment of Foot Corns
Categorical or Qualitative Data. Nominal Categorical Data
Ordinal Data
Ranks
Numerical or Quantitative Data. Count Data
Measured or Numerical Continuous
Interval and Ratio Scales
2.2 Summarising Categorical Data
Illustrative Example – Salicylic Acid Plasters for Treatment of Foot Corns
Labelling Binary Outcomes
2.3 Displaying Categorical Data
2.4 Summarising Continuous Data
Measures of Location – The Three ‘Ms’ – Mean, Median and Mode. Mean or Average
Example – Calculation of the Mean – Corn Size Data (mm)
Median
Example – Calculation of the Median – Corn Size Data
Mode
Example – Calculation of the Mode – Corn Size Data
Measures of Dispersion or Variability
Range
Example – Calculation of the Range – Corn Size Data
Quartiles and the Interquartile Range
Percentiles
Example – Calculation of the Range, Quartiles, and Inter‐Quartile Range – Corn Size Data
Standard Deviation and Variance
Illustrative Example – Calculation of the Standard Deviation – Foot Corn Size
Why is the Standard Deviation Useful?
Means or Medians?
2.5 Displaying Continuous Data
Dot Plots
Example – Dot Plot – Baseline Corn Size
Histograms
Box and Whisker Plot
Illustrative Example – Box and Whisker Plot – Birthweight by Type of Delivery
Scatter Plots
Illustrative Example – Scatter Plot – Baseline Corn Size by Corn Size at a Three month Follow‐up
Measures of Symmetry
2.6 Within‐Subject Variability
Illustrative Example – Within‐Subject Variability – Total Steps per Day
2.7 Presentation. Graphs
Tables
2.8 Points When Reading the Literature
2.9 Technical Details. Calculating the Sample Median
Calculating the Quartiles and Inter Quartile Range
2.10 Exercises
3 Summary Measures for Binary Data
Summary
3.1 Summarising Binary and Categorical Data
Ratios, Proportions, Percentages, Risk and Rates
Illustrative Example – RCT of Salicylic Acid Plasters for Treatment of Foot Corns
Labelling Binary Outcomes
Comparing Outcomes for Binary Data
Summarising Comparative Binary Data – Differences in Proportions
Example – Summarising Results from a Clinical Trial – Corn Plasters RCT: Differences in Proportions
Summarising Comparative Binary Data – Relative Risk
Example – Summarising Results from a Clinical Trial – Corn Plasters RCT: Relative Risk
Summarising Comparative Binary Data – Number Need to Treat
Example – Summarising Results from a Clinical Trial – Corn Plasters RCT: NNT
Issues with NNT – Always Consider all the Risks
Example – Importance of Considering both Absolute Risk and Relative Risk
Summarising Binary Data – Odds and Odds Ratios
Example – Summarising Results from a Clinical Trial– Corn Plasters: Odds Ratio
Why Should One Use the Odds Ratio?
The Odd Ratios are Symmetrical but the Relative Risk Is Not
How Are Risks Compared?
3.2 Points When Reading the Literature
3.3 Exercises
4 Probability and Distributions
Summary
4.1 Types of Probability
Examples of Addition and Multiplication Rules – Using Dice Rolling
Probability Distributions for Discrete Outcomes
4.2 The Binomial Distribution
Example – Probability of Corn Resolving
4.3 The Poisson Distribution
Example from the Literature – IV Treated Exacerbations in Patients with Cystic Fibrosis
4.4 Probability for Continuous Outcomes
4.5 The Normal Distribution
How Do We Use the Normal Distribution?
Illustrative Example – Normal Distribution – Birthweights
4.6 Reference Ranges
Worked Example – Reference Range – Birthweight
4.7 Other Distributions
t‐distribution
Chi‐squared Distribution
F‐distribution
Uniform Distribution
4.8 Points When Reading the Literature
4.9 Technical Section. Binomial Distribution
Poisson Distribution
Normal Distribution
4.10 Exercises
5 Populations, Samples, Standard Errors and Confidence Intervals
Summary
5.1 Populations
5.2 Samples
5.3 The Standard Error. Standard Error of the Mean
Properties of the Distribution of Sample Means
Properties of Standard Errors
Worked Example – Standard Error of a Mean – Birthweight of Preterm Infants
5.4 The Central Limit Theorem
5.5 Standard Errors for Proportions and Rates
Worked Example – Standard Error of a Proportion – Mortality from Gastrostomy in Patients with Amyotrophic Lateral Sclerosis (ProGas): A Prospective Cohort Study (ProGas Study Group 2015)
Worked Example – Standard Error of a Rate – Exacerbations in Patients with Cystic Fibrosis
Standard Deviation or Standard Error?
5.6 Standard Error of Differences
Worked Example – Standard Error of Difference in Means – Corn Size
Worked Example – Difference in Proportions –Haemorrhoid Recurrence at One Year (HubBLe Trial)
5.7 Confidence Intervals for an Estimate. Confidence Interval for a Mean
Worked Example – Confidence Interval for a Mean – Birthweights of Pre‐term Infants
Confidence Interval for a Proportion
Example from the Literature – Confidence Interval for a Proportion –Gastrostomy in Patients with Amyotrophic Lateral Sclerosis (ProGas)
Confidence Interval for a Rate
Worked Example – Confidence Interval for a Rate – Exacerbations in Patients with Cystic Fibrosis
5.8 Confidence Intervals for Differences
Example from the Literature – Confidence Interval for the Difference Between Two Means – Corn Size at Three Months
Worked Example – Confidence Interval for Difference Between Two Proportions – Haemorrhoid Recurrence at One Year (HubBLe Trial)
5.9 Points When Reading the Literature
5.10 Technical Details. Standard Errors
More Accurate Confidence Intervals for a Proportion
Worked Example – Confidence Interval for a Proportion – Gastrostomy in Patients with Amyotrophic Lateral Sclerosis (ProGas)
5.11 Exercises
6 Hypothesis Testing, P‐values and Statistical Inference
Summary
6.1 Introduction
6.2 The Null Hypothesis
Example: Distance Walked On an Endurance Shuttle Walking Test (ESWT) Before and After a Rehabilitation Programme in Patients with COPD (Waterhouse et al. 2010)
6.3 The Main Steps in Hypothesis Testing
Step 1: State Your Null Hypothesis (H0) and Alternative Hypothesis (HA)
Step 2: Choose a Significance Level, α, for Your Test
Step 3: Obtain the Probability of Observing Your Results, or Results More Extreme, if the Null Hypothesis is True (P‐value)
Step 4: Use Your P‐value to Make a Decision About Whether to Reject, or Not Reject, Your Null Hypothesis
Example: Distance Walked on a 6MWT Before and After a Rehabilitation Programme
Step 1: State Your Null Hypothesis (H0) and Alternative Hypothesis (HA)
Step 2 Choose a Significance Level, α, for Your Test
Step 3 Obtain the Probability of Observing Your Results, or Results More Extreme, If the Null Hypothesis is True (P‐value)
6.4 Using Your P‐value to Make a Decision About Whether to Reject, or Not Reject, Your Null Hypothesis
Example – Interpreting a P‐value – Distance Walked Before and After Exercise
What Does P< 0.001 Mean?
Is This Result Statistically Significant?
You Decide?
Summary of the Main Steps in Hypothesis Testing
6.5 Statistical Power. Type I Error, Test Size and Significance Level
Type II Error and Power
6.6 One‐sided and Two‐sided Tests
6.7 Confidence Intervals (CIs)
Example – Clinical Importance – Change in Distance Walked Before and After Exercise
Relationship Between Confidence Intervals and Statistical Significance
Clinically Important Difference
6.8 Large Sample Tests for Two Independent Means or Proportions
Large Sample Z‐Test for Comparison of Two Independent Means
Z‐test for Comparison of Two Independent Proportions
Example from the Literature – Haemorrhoidal Artery Ligation Versus Rubber Band Ligation for the Management of Symptomatic Second‐degree and Third‐degree Haemorrhoids (HubBLe Trial)
6.9 Issues with P‐values
6.10 Points When Reading the Literature
6.11 Exercises
7. Comparing Two or More Groups with Continuous Data
Summary
7.1 Introduction
7.2 Comparison of Two Groups of Paired Observations – Continuous Outcomes
Box 7.1 Paired t‐Test
Assumptions
Steps
Wilcoxon (Matched Pairs) Signed Rank Test
Box 7.2 Wilcoxon (Matched Pairs) Signed Rank Test
Assumptions
Steps
7.3 Comparison of Two Independent Groups – Continuous Outcomes
Box 7.3 Independent Two‐Sample t‐Test for Comparing Means
Assumptions
Steps
Mann–Whitney U Test
Box 7.4 Mann–Whitney U Test
Assumptions
Steps
Discrete Count Data
7.4 Comparing More than Two Groups
One‐way Analysis of Variance
The Kruskal–Wallis Test
7.5 Non‐Normal Distributions. Non‐parametric Tests
Why Not Always Use Non‐parametric Tests?
7.6 Degrees of Freedom
7.7 Points When Reading the Literature
7.8 Technical Details. Student's t‐Distribution
Welch's t‐Test
Wilcoxon Signed Rank Sum Test
Mann–Whitney U Test
Comparing More than Two Groups. One‐way Analysis of Variance
Box 7.5 One‐way Analysis of Variance (ANOVA)
Assumptions
Steps
The Kruskal–Wallis Test
Box 7.6 Kruskal–Wallis Test
Assumptions
Steps
7.9 Exercises
8 Comparing Groups of Binary and Categorical Data
Summary
8.1 Introduction
8.2 Comparison of Two Independent Groups – Binary Outcomes
Box 8.1 Chi‐squared Test for Association in r × c Contingency Tables
Assumptions
Steps
Fisher's Exact Test
Chi‐squared Test for Trend in a 2 × c Table
8.3 Comparing Risks
Comparing Groups Via the Odds Ratio
8.4 Comparison of Two Groups of Paired Observations – Categorical Outcomes
8.5 Degrees of Freedom
8.6 Points When Reading the Literature
8.7 Technical Details. Fisher's Exact Test
Worked Example: Calculation of Fisher's Exact Test
Chi‐squared Test for Trend (2 × c Table)
Chi‐squared Test for Trend in 2 × c Contingency Tables
Assumptions
Steps
Exact Test for Small Samples with Paired Binary Outcomes
Example
Confidence Interval for a Relative Risk
Worked Example
CI for an Odds Ratio
Worked Example
8.8 Exercises
9 Correlation and Linear Regression
Summary
9.1 Introduction
Example – Systolic and Diastolic Blood Pressure
9.2 Correlation
Box 9.1 Pearson's Correlation Coefficient
Assumptions
Steps
Hypothesis test for ρ
Confidence interval for ρ
When Not to Use the Correlation Coefficient
Example – Correlation Between Systolic and Diastolic Blood Pressure
Assumptions Underlying the Test of Significance
Box 9.2 Spearman's Rank Correlation Coefficient
Assumptions
Steps
Example – Spearman and Pearson Correlation Coefficients – Systolic and Diastolic Blood Pressure
9.3 Linear Regression. The Regression Line
Box 9.3 Linear Regression
Assumptions
Steps
Example – Linear Regression – Birthweight and Gestation
Tests of Significance and Confidence Intervals
Example – Linear Regression – Birthweight and Gestational Age – Hypothesis Test and Confidence Interval
Do the Assumptions Matter?
Regression and Prediction
Example – Prediction – Birthweight
9.4 Comparison of Assumptions Between Correlation and Regression
9.5 Multiple Regression. The Multiple Regression Equation
Examples of Uses of Multiple Regression
9.6 Correlation is not Causation
Association Between Two Variables: Correlation or Regression?
9.7 Points When Reading the Literature
9.8 Technical Details
Correlation Coefficient. Worked Example – Correlation Coefficient
Spearman's Rank Correlation
Linear Regression
Worked Example – Linear Regression
Worked Example – Calculation of Residuals
Technical Details: Checking the Assumptions for a Linear Regression Analysis
9.9 Exercises
10 Logistic Regression
Summary
10.1 Introduction
10.2 Binary Outcome Variable
Example – Logistic Regression – Foot Corn RCT
10.3 The Multiple Logistic Regression Equation
Example – Multiple Logistic Regression – Corn Healed and Baseline Corn Size
Calculating the Probability of an Event
Example – Estimating the Probability that an Individual Has the Outcome of Interest – Corning Healing
Example of Multiple Logistic Regression – Foot Corn RCT
Use of Logistic Regression in Case–Control Studies
Example from the Literature – Caffeinated Substances and Risk of Crashes in Long Distance Drivers: Case–Control Study
10.4 Conditional Logistic Regression
Example of Conditional Logistic Regression
Consequences of the Logistic Model
Example – Foot Corn RCT Group by Sex Interaction
Model Checking
10.5 Reporting the Results of a Logistic Regression
10.6 Additional Points When Reading the Literature When Logistic Regression Has Been Used
10.7 Technical Details. The Logistic Regression Model
10.8 The Wald Test
10.9 Evaluating the Model and its Fit: The Hosmer–Lemeshow Test
10.10 Assessing Predictive Efficiency (1): 2 × 2 Classification Table
10.11 Assessing Predictive Efficiency (2): The ROC Curve
10.12 Investigating Linearity
10.13 Exercises
11 Survival Analysis
Summary
11.1 Time to Event Data
Example from the Literature – Trial Endpoints – Children with Neuroblastoma
Censored Observations Can Arise in Three Ways
11.2 Kaplan–Meier Survival Curve
Example from the Literature – Kaplan–Meier Curves – Aim High Study
Procedure for Calculating a Kaplan–Meier Survival Curve
Worked Example – K‐M Survival Curve
11.3 The Logrank Test
Procedure for Calculating the Logrank Test Comparing Two Groups A and B
Worked Example – Logrank Test
11.4 The Hazard Ratio
Worked Example – Median ‘Survival’ Time – AIM High
Procedure for Calculating the HR when Comparing Two Groups
Worked Example – Hazard Ratio – 20 Randomly Sampled Patients from the AIM High Trial
Confidence Interval for HR
95% Confidence Interval (CI) for an HR
Worked Example – Confidence Interval for the HR – AIM High Trial
11.5 Modelling Time to Event Data
Cox Proportional Hazards Regression Model for Two Groups and No Covariates
Technical Example
Worked Example – Cox Model
Example from the Literature – Cox Multivariable Regression – AIM High Trial
11.6 Points When Reading Literature. Interpreting the Results of a Survival Analysis
Technical Details
11.7 Exercises
12 Reliability and Method Comparison Studies
Summary
12.1 Introduction
12.2 Repeatability
Coefficient of Variation
Coefficient of Variation
Example – CV – Total Steps Per Day
Intra‐class Correlation Coefficient (ICC)
Intra‐Class Correlation Coefficient
Example from the Literature – ICC – Paediatric Asthma Quality of Life Questionnaire
Comparison Between the Intraclass Correlation and Pearson's Correlation
12.3 Agreement
Observer(s) Agreement
Observer(s) Disagreement
Cohen's Kappa (κ)
Cohen's κ
Example from the Literature – Cohen's κ – Arteriovenous Malformations (AVM)
Worked Example – Cohen's κ – Pathology Review
12.4 Validity. Cronbach's Alpha (αCronbach)
Cronbach's Alpha, αCronbach
Example from the Literature – αCronbach – Patients with Pressure Ulcers (PU)
12.5 Method Comparison Studies
Example – Method Comparisons – Two Spirometers
Why Calculating the Correlation Coefficient is Inappropriate
Example – Bland and Altman Plots – Comparing Respiradyne and Vitalograph Spirometers
Method Comparisons
Example – Limits of Agreement – Two Spirometers
Example from the Literature – Limits of Agreement – Positron Emission Tomography (PET) and Endoscopic Ultrasound (EUS)
12.6 Points When Reading the Literature
12.7 Technical Details
Agreement by Chance (PChance)
Calculation of Cronbach's Alpha
12.8 Exercises
13. Evaluation of Diagnostic Tests
Summary
13.1 Introduction
13.2 Diagnostic Tests
13.3 Prevalence, Overall Accuracy, Sensitivity, and Specificity
Example of Calculations for Sensitivity and Specificity – Diagnosis of Prostate Cancer (Ahmed et al. 2017 )
13.4 Positive and Negative Predictive Values
Example of Calculations for PPV and NPV – Diagnosis of Prostate Cancer (Ahmed et al. 2017 )
13.5 The Effect of Prevalence
13.6 Confidence Intervals
13.7 Functions of a Screening and Diagnostic Test
13.8 Likelihood Ratio, Pre‐test Odds and Post‐test Odds
Example – Likelihood Ratio – MRI Scan for Diagnosing Prostate Cancer
13.9 Receiver Operating Characteristic (ROC) Curve
Example of Calculations – Diagnosis of PND Using the EPDS
Example from the Literature – ROC – Diagnosis of Postnatal Depression Using the EPDS
Analysis of ROC Curves
Discussion
13.10 Points When Reading the Literature About a Diagnostic Test
Technical Details. Bayes' Theorem
Example: Conditional Probabilities and Diagnostic Testing
13.11 Exercises
14 Observational Studies
Summary
14.1 Introduction
14.2 Risk and Rates
Risk is Defined as:
To Calculate a Rate the Following Are Needed
Incidence is Defined as:
Crude Mortality Rate
Example of Crude Mortality Rates
Age‐Specific Rates (ASRs)
Age‐Specific Rate
Direct and Indirect Age‐Standardised Rates
Standardised Mortality Ratio (SMR)
Issues in the Use of Standardisation
Incidence and Prevalence
14.3 Taking a Random Sample
14.4 Questionnaire and Form Design. Purpose of Questionnaires and Forms
Types of Questions
14.5 Cross‐sectional Surveys
Example from the Literature – Prevalence of Asthma in Greece
14.6 Non‐randomised Studies. Pre‐test/Post‐test Studies
Example from the Literature – Before‐and‐After Studies
Example from the Literature – Observational Study – Acute Myocardial Infarction
Quasi‐experimental Designs
Example from the Literature – Quasi‐experimental Study – Cancer in Gulf War Veterans
Interrupted Time Series
Example of an Interrupted Time Series
14.7 Cohort Studies
Design
Relative Risk
Example from the Literature – Cohort Study – Mortality in Slate Workers
The Population Attributable Risk
Attributable Risk
Worked Example – Attributable Risk
Why Quote a Relative Risk?
Size of Study
Problems in Interpretation of Cohort Studies
Post‐marketing Surveillance
14.8 Case–Control Studies. Design
Unmatched Study
Example from the Literature – Case–Control Study – Mobile Phone Use and Glioma
Odds Ratio
Worked Example – Odds Ratio – Mobile Phone Use and Glioma
Matched Studies
Odds Ratio for a Matched Case–Control Study
Example from the Literature – Matched Case Control Study – Adverse Childhood or Adult Experiences and Risk of Bilateral Oophorectomy
Analysis by Matching?
Selection of Controls
Confounding
Example from the Literature – Simpson's Paradox
Limitations of Case–Control Studies
14.9 Association and Causality
14.10 Modern Causality Methods and Big Data
14.11 Points When Reading the Literature
14.12 Technical Details. Confidence Interval for a Relative Risk
Worked Example
CI for an Odds Ratio
Worked Example
CI for Matched Case Control Study
Worked Example
14.13 Exercises
15 The Randomised Controlled Trial
Summary
15.1 Introduction
15.2 The Protocol
15.3 Why Randomise? Randomisation
Random Assignment and the Protocol
Historical Controls
15.4 Methods of Randomisation. Simple Randomisation
Blocked Randomisation
Stratified Randomisation
Carrying out Randomisation
15.5 Design Features. The Need for a Control Group
Treatment Choice and Follow‐up
Blind Assessment
Pilot Trials and Feasibility Studies
Design Features of Feasibility Studies and Pilot Trials
Example – External Pilot Trial – Physiotherapy Management of Lumbar Radicular Syndrome (LRS) – The POLAR Trial
Example – External Pilot Trial – Early Pulmonary Rehabilitation (EPR) in Patients with Chronic Obstructive Pulmonary Disease (COPD)
Example – Internal Pilot Trial – Endometrial Trauma in Women Undergoing in vitro Fertilisation (IVF)
Protocol Violations
‘Pragmatic’ and ‘Explanatory’ Trials
Superiority and Equivalence Trials
Design Features of Equivalence Trials
Example – Non‐inferiority – Adjuvant Treatment of Postmenopausal Women with Early Breast Cancer
Early and Late Phase Trials
15.6 Design Options. Parallel Designs
Example from the Literature – Parallel Group Trial – Reduced Nicotine Cigarettes
Cross‐over Designs
Example from the Literature – Cross‐over Trial – Nutrition in Diabetes
Cluster Randomised Controlled Trials
Example from the Literature – Cluster Design – Diabetes
Stepped Wedge Cluster Randomised Trials
Example for the Literature – Stepped Wedge Cluster Randomised Trial – SHAREHD
Factorial Trials
Example from the Literature – 2 × 2 Factorial Design – AspECT Trial
15.7 Meta‐analysis
15.8 Checklists for Design, Analysis and Reporting
Design
Checklist of Design Features
Analysis and Presentation
Checklist for Analysis and Presentation
15.9 CONSORT
15.10 Points When Reading the Literature About a Trial
15.11 Exercises
16 Sample Size Issues
Summary
16.1 Introduction. Why Sample Size Calculations?
Why Not Sample Size Calculations?
Summing Up
16.2 Study Size
Fundamental Ingredients for a Sample Size Calculation
Type I and Type II Error Rates
Standard Deviation of the Outcome Measure
Tips on Finding the Anticipated Standard Deviation of an Estimate
Control Group Response
The (Anticipated) Effect Size
Relationship Between Type I Error, Type II Error and Effect Size
Directions of Sample Size Estimates
16.3 Continuous Data
Worked Example – Simple Formula for a Continuous Variable – Behavioural Therapy
Example from the Literature of a Sample Size for a Continuous Outcome – The IPSU Trial
16.4 Binary Data
Example from the Literature of Sample Size Calculation for Binary Outcomes – Corn Plaster Trial
16.5 Prevalence
Worked Example – Sample Size – Prevalence
16.6 Subject Withdrawals
Example from the Literature – Withdrawals – The IPSU Trial
16.7 Other Aspects of Sample Size Calculations. Pilot Studies
Example from Literature of Sample Size for a Pilot or Feasibility Study – The POLAR Trial
Post‐hoc Sample Size Calculations
Other Designs
Summary
16.8 Points When Reading the Literature
16.9 Technical Details
Continuous Outcomes – Comparison of Two Means
Worked Example – Comparison of Two Means
Binary Outcomes – Comparison of Two Proportions
Worked Example – Comparison of Two Proportions
16.10 Exercises
17 Other Statistical Methods
Summary
17.1 Analysing Serial or Longitudinal Data
Three Broad Approaches to Analysing Repeated Measurements
Summarising Repeated Measurements
Time by Time Analysis
Response Feature Analysis – The Use of Summary Measures
The Area Under the Curve (AUC)
Calculating the AUC
Example from the Literature – AUC and the POLAR Trial
Other Summary Measures
Longitudinal Models
17.2 Poisson Regression
Use of an Offset
Calculating the Probability of an Event
Example from the Literature – Cystic Fibrosis the ACTif Trial
Extra‐Poisson Variation
17.3 Missing Data
Types and Patterns of Missing Data
Describing the Extent and Patterns of Missing Data
Incomplete Data
What if we Think the Data Is MNAR?
Imputation of Missing Data
Example – Imputation of Missing Data – IPSU Trial
17.4 Bootstrap Methods
A Simple Example of the Bootstrap
Bootstrap Methods for Confidence Interval Estimation
Example from the Literature
17.5 Points When Reading the Literature
17.6 Exercises
18 Meta‐analysis
Summary
18.1 Introduction
18.2 What is a Meta‐analysis?
Box 18.1 Meta‐analysis: What Does it Achieve?
18.3 Meta‐analysis Methods. Is a Meta‐analysis Appropriate? The PICOTS Approach
Fixed Effects and Random Effects Meta‐analysis
Combining the Results of Different Studies
Heterogeneity
18.4 Example: Mobile Phone Based Intervention for Smoking Cessation
18.5 Discussion. The Main Problems with Meta‐analysis
Presentation of Results
Further Reading
18.6 Technical Details. Fixed Effects
Random Effects
18.7 Exercises
19 Common Mistakes and Pitfalls
Summary
19.1 Introduction
19.2 Misleading Graphs and Tables. Two‐ or Three‐Dimensional Charts
Dynamite Plunger Plots
Supress the Origin or Change the Baseline
Presenting Data in Tables
19.3 Plotting Change Against Initial Value. Adjusting for Baseline
Worked Example – Weight Change
Example – Weight Change
Regression to the Mean
Example from the Literature – Change in Fasting Serum Cholesterol
19.4 Within Group Versus Between Group Analyses
14.4 P‐value from Paired t‐test
P‐value from Two Independent Samples t‐test
19.5 Analysing Paired Data Ignoring the Matching
19.6 Unit of Analysis
19.7 Testing for Baseline Imbalances in an RCT
19.8 Repeated Measures
Example – Postprandial Plasma Glucose Concentrations
Invalid Approaches
Example – Mean Response Over Time
Valid Approaches
Example – Liraglutide Versus Sitagliptin for Type 2 Diabetes
19.9 Clinical and Statistical Significance
19.10 Post Hoc Power Calculations
19.11 Predicting or Extrapolating Beyond the Observed Range of Data
19.12 Exploratory Data Analysis ‘Fishing Expeditions’
Multiple Comparisons
19.13 Misuse of P‐values
19.14 Points When Reading the Literature
Appendix Statistical Tables
Solutions to Multiple‐Choice Exercises. Chapter 2
Chapter 3
Chapter 4
Chapter 5
Chapter 6
Chapter 7
Chapter 8
Chapter 9
Chapter 10
Chapter 11
Chapter 12
Chapter 13
Chapter 14
Chapter 15
Chapter 16
Chapter 17
Chapter 18
References (Chapter numbers in square brackets)
Index. a
b
c
d
e
f
g
h
i
k
l
m
n
o
p
q
r
s
t
u
v
w
y
z
WILEY END USER LICENSE AGREEMENT
Отрывок из книги
Fifth Edition
Stephen J. Walters
.....
Table 2.5 Calculating the median, quartiles, and interquartile range for the corn size data.
The variance is expressed in square units and so is not a suitable measure for describing variability because it is not in the same units as the raw data. The solution is to take the square root of the variance to return to the original units. This gives us the standard deviation (usually abbreviated to SD or s) defined as:
.....